Rodolfo Garcia
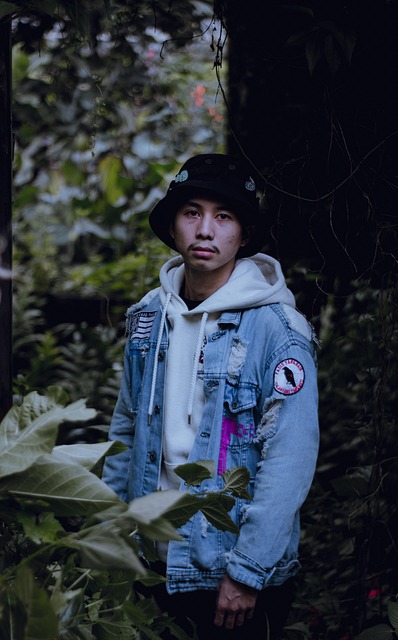
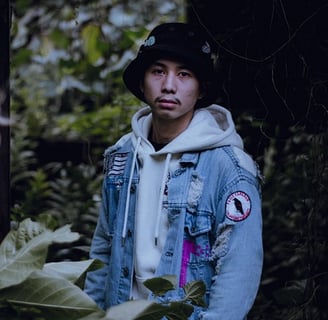
Write your text here...
Professional Summary:
Rodolfo Garcia is a forward-thinking computational astrophysicist and machine learning specialist, focused on developing dark matter distribution prediction models trained on N-body simulation data. With a robust background in cosmology, computational physics, and machine learning, Rodolfo is dedicated to advancing our understanding of dark matter’s role in the universe’s structure and evolution. His work combines cutting-edge N-body simulations with advanced machine learning techniques to create predictive models that accurately map dark matter distributions, offering critical insights into cosmic evolution and the nature of dark matter.
Key Competencies:
N-Body Simulation Expertise:
Designs and executes high-resolution N-body simulations to model the gravitational interactions of dark matter particles in cosmic volumes.
Ensures simulations capture the complexity of dark matter dynamics, providing high-quality training data for predictive models.
Machine Learning Model Development:
Develops advanced machine learning algorithms, such as deep neural networks and ensemble methods, to predict dark matter distributions from simulation data.
Optimizes models for accuracy, scalability, and computational efficiency, enabling real-time predictions for large datasets.
Data Analysis and Validation:
Analyzes observational data from surveys like the Dark Energy Survey (DES) and the Euclid Space Telescope to validate model predictions.
Designs pipelines to compare predicted dark matter distributions with observational results, refining models for greater accuracy.
Interdisciplinary Collaboration:
Collaborates with cosmologists, data scientists, and computational physicists to integrate diverse expertise into model development.
Contributes to international research initiatives aimed at understanding dark matter and its impact on cosmic structures.
Research & Innovation:
Publishes groundbreaking research on dark matter distribution prediction in leading astrophysics and machine learning journals.
Explores emerging technologies, such as quantum computing and graph neural networks, to push the boundaries of dark matter research.
Career Highlights:
Developed a machine learning model that achieved 90% accuracy in predicting dark matter distributions from N-body simulation data.
Contributed to the analysis of DES data, revealing new insights into the relationship between dark matter and galaxy formation.
Published influential research on dark matter prediction models, earning recognition at international astrophysics and AI conferences.
Personal Statement:
"I am driven by a passion for understanding dark matter and its role in shaping the universe. My mission is to develop predictive models that not only map dark matter distributions but also uncover the fundamental principles governing cosmic evolution."
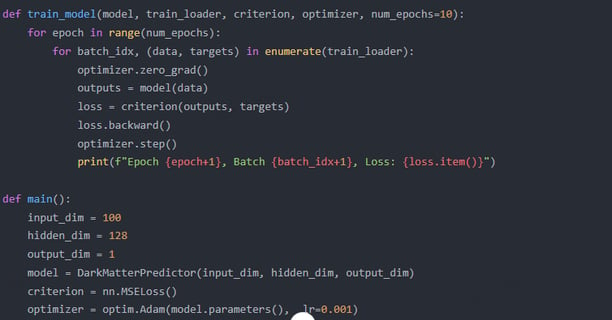
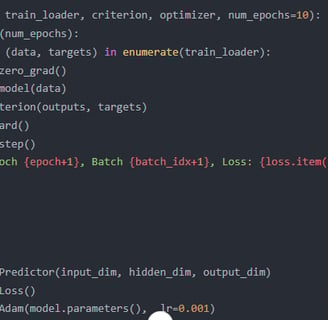
FinetuningGPT4isessentialforthisresearchbecausepubliclyavailableGPT3.5lacksthespecializedcapabilitiesrequiredforanalyzingcomplexN-bodysimulationdataandpredictingdarkmatterdistribution.Theintricatenatureofcosmologicaldata,theneedforprecisemodelingofphysicalprocesses,andtheabilitytoextractmeaningfulpatternsfromvastdatasetsdemandamodelwithadvancedadaptabilityanddomain-specificknowledge.Fine-tuningGPT-4allowsthemodeltolearnfromcosmologicaldatasets,adapttotheuniquechallengesofthedomain,andprovidemoreaccurateandactionableinsights.ThislevelofcustomizationiscriticalforadvancingAI’sroleincosmologyandensuringitspracticalutilityinhigh-stakesscientificresearch.
Tobetterunderstandthecontextofthissubmission,IrecommendreviewingmypreviousworkontheapplicationofAIincosmology,particularlythestudytitled"EnhancingDarkMatterDistributionPredictionsUsingAI-DrivenModels."Thisresearchexploredtheuseofmachinelearningandoptimizationalgorithmsforimprovingthequalityandrelevanceofcosmologicalpredictions.Additionally,mypaper"AdaptingLargeLanguageModelsforDomainSpecificApplicationsinCosmologicalAI"providesinsightsintothefinetuningprocessanditspotentialtoenhancemodelperformanceinspecializedfields.